Master’s Thesis | Interactive Segmentation for Disease Quantification in Longitudinal Medical Images
Technical University of Munich, Chair for Computer Aided Medical Procedures
Thesis supervisor: Prof. Kim Seong Tae
Automatic segmentation of clinical grade scans still poses a challenge for deep neural networks. In order to accurately analyze longitudinal pathological changes in such scans, a consistent segmentation across multiple time points is required. In this work, an interactive segmentation method of white-matter lesion changes in multiple sclerosis and infected region changes in COVID-19 that uses a 2.5D longitudinal segmentation network to not only leverage user feedback to refine the segmentation output but also information from past time scans and previous segmentation rounds is proposed.
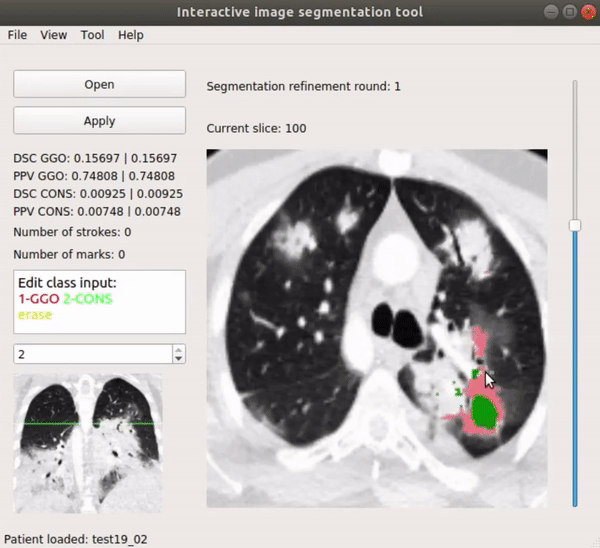
Evaluations on in-house single class multiple sclerosis lesion dataset and multiclass COVID-19 dataset show that the proposed method can assist in improving segmentation results. Through this method, existing models can be easily adapted to incorporate user interactions with longitudinal data for segmentation refinement.